Neural Networks Prove To Be a Key Technique in Analyzing Gravitational Lenses
Credit to Author: Michael Byrne| Date: Fri, 01 Sep 2017 12:15:40 +0000
Physicists at Stanford University have developed a new technique of using neural networks for analyzing gravitational lenses in distant space. The method is some 10 million times faster than current strategies, offering a key upgrade to our ability to make sense from observations of light from far-flung galaxies.
The problem is that in peering out into space, we don’t really see things as they are. Here in my office I can be reasonably sure that my coffeecup is where it is and my dog isn’t where he isn’t, but at these scales I don’t really have to worry about the intervening effects of gravity on my perspective. When it comes to space, I do. Per relativity, gravity bends light, often dramatically so.
A key manifestation of this is known as gravitational lensing. If I have one galaxy that is very far away and it’s the only galaxy around, I can imagine that I have a clear view of it. But if there’s something with a lot of mass in between me and it, like a black hole or cluster of stars, then that intervening object is going to distort the light from the galaxy. This is the basic idea of a gravitational lens―a massive stellar body whose gravity serves to focus distant light. When it comes to observing distant light sources, this is often a very beneficial phenomenon, like having a natural telescope.
Finding gravitational lenses is tricky. Generally, it’s a process of taking observed images and matching them to computer models, which sounds easy enough but can actually take several weeks per lens and requires enormous computing resources. Compounding the problem is the fact that the next generation of telescopes is about to introduce a deluge of gravitational lens discoveries in the very near future. We can expect tens of thousands of new lenses and their associated data requiring analysis.
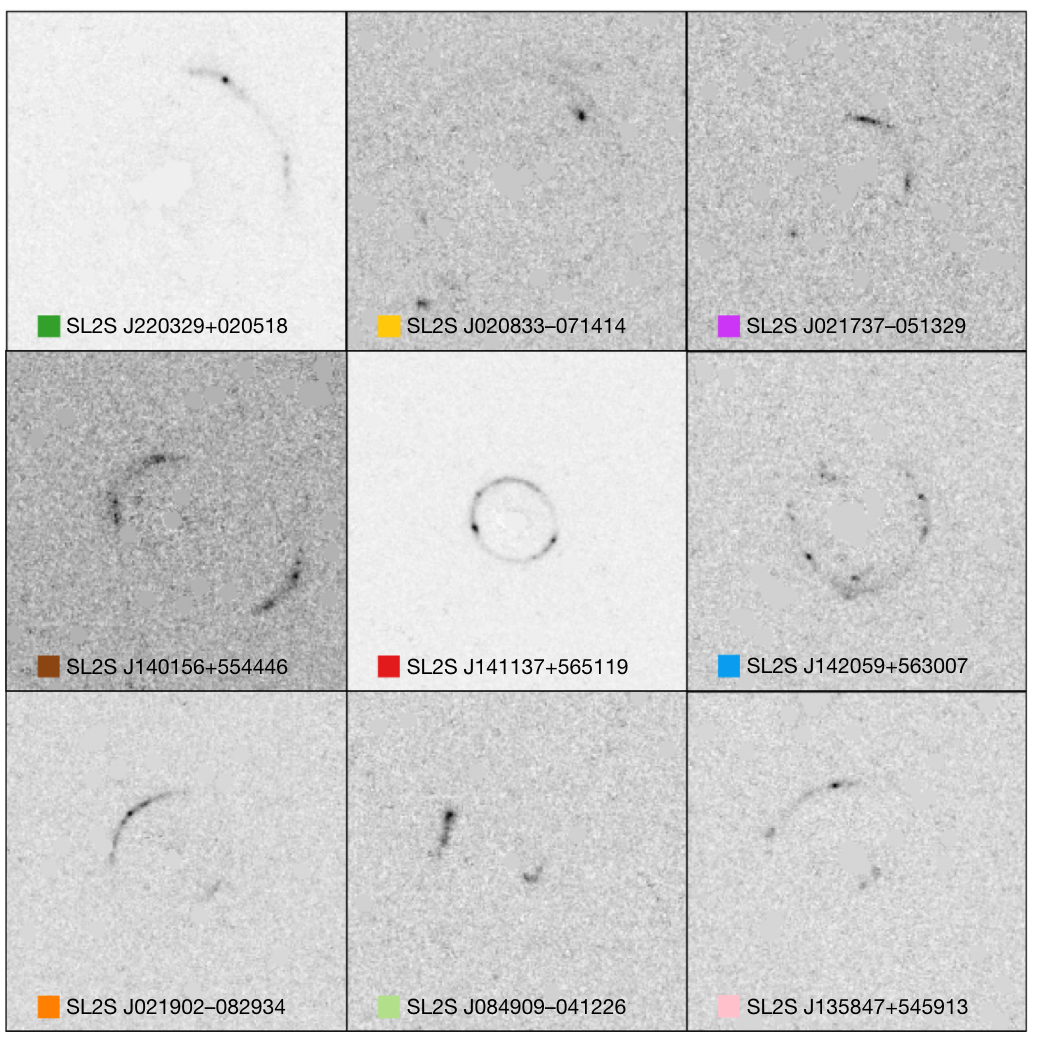
The utility of a neural network (and machine learning, in general) is in its ability to make generalizations from large data sets. Show a learning algorithm enough examples of a certain phenomenon and it should eventually be able to identify that phenomenon in new, unseen data.
Previous research has already demonstrated that it’s possible to identify gravitational lenses like this, but the current work goes further, demonstrating that it’s possible to characterize the gravitational lenses as well. Via neural network classification, the Stanford researchers were able to calculate gravitational lens parameters like ellipticity, Einstein radius, and lens center.
What’s more, we can expect this technique to extend to the characterization of other sorts of cosmic bodies as well. “Neural networks provide a fast alternative to the maximum likelihood methods that are commonly used to estimate parameters of interest in astrophysics from imaging data,” the Stanford group concludes. “Their effectiveness for image analysis makes them a powerful tool for applications beyond lensing studies.”
In the near future, we simply won’t have the manpower to parse all of the cosmic data that will be flooding in from new telescopes. Neural networks offer an automated way in.
“Neural networks will help us identify interesting objects and analyse them quickly,” study co-author Perreault Levasseur told Physics World. “This will give us more time to ask the right questions about the universe.”